Imaging of Matter
Machine learning enables counting of magnetic skyrmions
16 May 2022
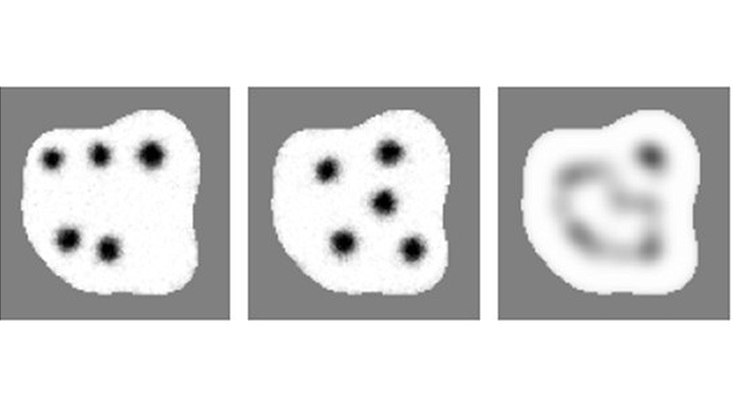
Photo: UHH, Tim Matthies
Researchers from the Department of Physics have succeeded in training artificial neural networks to reconstruct the skyrmion number in confined geometries. In the journal Physical Review Applied, they report how the topological charge can be determined from a time-averaged measurement in this way. The results are of immediate importance for the interpretation of experimental results, for skyrmion-based computing, and for memory concepts.
Skyrmions are local whirls in the spin texture of a magnetic material. Magnetic interactions at the atomic level can give rise to various magnetic structures. The magnetic skyrmion is a particularly interesting configuration in this context: recently proposed spintronic devices use magnetic skyrmions as bits of information. "However, in order to use magnetic skyrmions, we need to be able to reliably detect them," says Tim Matthies from the research groups of Prof Roland Wiesendanger (subgroup Dr Elena Y. Vedmedenko), and Dr Thore Posske, both conducting research as parts of the cluster of excellence "CUI: Advanced Imaging of Matter".
High mobility makes accurate measurement difficult
For well-separated skyrmions, the topological charge corresponds to the number of skyrmions in the system. However, the high mobility of magnetic skyrmions leads to stochastic motion at finite temperatures, which makes accurate measurement of the topological numbers much more difficult.
To calculate the topological charge, researchers need the three-dimensional direction of each spin; however, only the z-direction of the spins is usually accessible in measurements. Complicating matters further, the skyrmions follow a Brownian motion at higher temperatures. Measuring the magnetization then yields a blurred image of the z-projection of the spins averaged over time, in which individual skyrmions are difficult to identify.
At this point, Tim Matthies and his colleague Alexander Schäffer employed an artificial neural network. "Surprisingly, using advanced machine learning techniques, one can extract the number of skyrmions even from this blurry data," says Tim Matthies. "This greatly facilitates skyrmion counting based on experimental real-space data."
Citation:
T. Matthies, A.F. Schäffer, T. Posske, R. Wiesendanger, and E.Y. Vedmedenko
“Topological characterization of dynamic chiral magnetic textures using machine learning”
Physical Review Applied
DOI: 10.1103/PhysRevApplied.17.054022