Imaging of Matter
Artificial intelligence identifies quantum phase transitions
8 July 2019
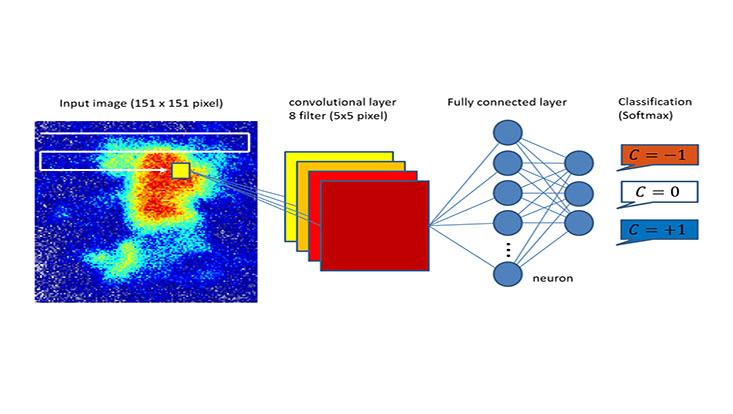
Photo: UHH/Sengstock group
Researchers from Universität Hamburg have used machine-learning techniques for improving the identification of quantum phase transitions from experimental data. To do so, they trained an artificial neural network to classify experimental images into one of the possible quantum phases. The researchers report on their findings in the journal Nature Physics.
Applications of artificial intelligence (AI) and machine learning are ranging from autonomous driving via fully automated industrial processes to daily life (‘intelligent refrigerator‘). These methods are also studied and used intensively in science, for example in particle physics, where relevant data are extracted from billions of data sets via AI-networks.
Now researchers from Hamburg have succeeded for the first time to apply AI in quantum physics for the identification of quantum phase transitions from experimental data, i.e. the point at which the properties of a substance change. This is particularly exciting, because the measurement via conventional analysis is much lengthier. The researchers stress that this result can have wide-ranging consequences for the daily research life. Artificial intelligence can analyze completely new quantum effects in real time in the laboratory, which are not accessible otherwise.
The team around Prof. Klaus Sengstock and Dr. Christof Weitenberg from the cluster of excellence “CUI: Advanced Imaging of Matter” and the SFB “Light-induced dynamics and control of correlated quantum systems” trained the AI by experimenting with gases cooled down to a few 100 nano-kelvin above absolute zero temperature of -273 degrees Celsius, so-called ultracold quantum gases. In the experiments, the researchers trap ultracold atoms in a lattice built from laser light and simulate the physics of electrons in solids.
If one changes the parameters of the lattices, the atoms arrange themselves differently and the gases get different properties. For example, while the gas conducts particles without friction in one phase, it isolates particles in another phase. The researchers are interested in the transitions between these phases, which differ in the measured momentum distribution. The team trained the neural network to assign the images obtained in the experiment to the correct phase and thus localize the phase transitions.
Weitenberg explains: „Previously, other researchers had demonstrated this approach for numerically generated images. That is also works with experimental data, is a promising result.“. Niklas Käming, a master student in the team who contributed substantially to the data analysis, adds: “The application of machine learning techniques to quantum gas experiments opens up many exciting possibilities. Next we want to extend the method to unsupervised machine learning, in which the images for the training do not need to be assigned to one of the phases.” Text: UHH, CUI
Citation:
Benno S. Rem, Niklas Käming, Matthias Tarnowski, Luca Asteria, Nick Fläschner, Christoph Becker, Klaus Sengstock, Christof Weitenberg
"Identifying Quantum Phase Transitions using Artificial Neural Networks on Experimental Data"
Nature Physics (2019)